- Analytics & Modeling - Computer Vision Software
- Analytics & Modeling - Machine Learning
- Education
- Equipment & Machinery
- Logistics & Transportation
- Product Research & Development
- Clinical Image Analysis
- Predictive Maintenance
- Cloud Planning, Design & Implementation Services
- Training
autoRetouch serves fashion image producers around the world, from retailers to editing services. These clients require high-quality images of their products for their online stores. The images often need edits such as background removal and skin retouching to ensure they accurately reflect the product being purchased. autoRetouch's clients are based all around the world, requiring the platform to be available around the clock. The platform's ability to scale from processing a few images to hundreds of thousands in seconds is crucial for these clients. The cost-effectiveness of the platform is also a significant factor, especially in the era of COVID-19, as companies shift their business online amid social distancing challenges.
autoRetouch, a Germany-based company, provides an automated image processing platform for fashion image producers worldwide. The shift to e-commerce has increased the demand for high-quality product images, which often require edits such as background removal and skin retouching. For retailers with hundreds of product lines, manually editing each image to perfection is a time-consuming and costly process. autoRetouch aimed to revolutionize this process by making it more time- and cost-effective. However, to train and run the models for its custom-developed machine learning algorithms, autoRetouch required powerful CPU and GPU processors that need to be available on demand. The platform had to be available around the clock and scale from processing a few images to hundreds of thousands, in seconds.
autoRetouch decided to partner with Google Cloud to overcome its challenges. The company used an open source Google TensorFlow project on semantic image segmentation called DeepLab as a starting point for its models. After a year of hard work and multiple iterations, autoRetouch achieved a Mean Intersection over Union (MIoU) score of 99.7% in benchmarking categories, indicating a very high level of accuracy. To access enough computational power to run these models cost-effectively, autoRetouch turned to Google Cloud. The company uses AI Platform to host its models and run online predictions using Google Kubernetes Engine, scaling CPU-based models automatically. It also uses Cloud Storage buckets for both its training data and output data. To train its power-intensive machine learning models, the company uses GPUs on Compute Engine.
Case Study missing?
Start adding your own!
Register with your work email and create a new case study profile for your business.
Related Case Studies.
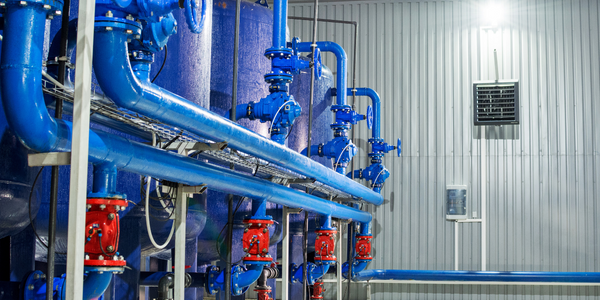
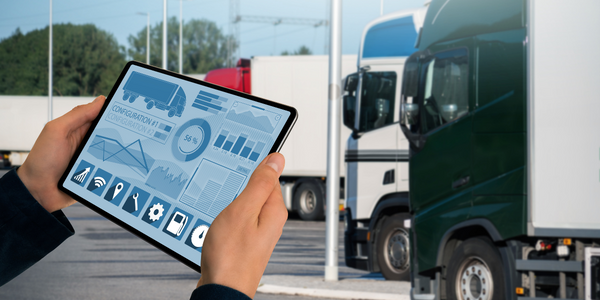
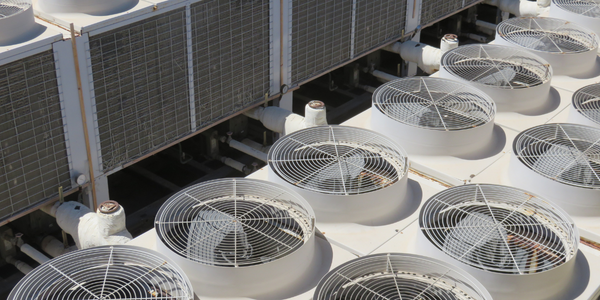
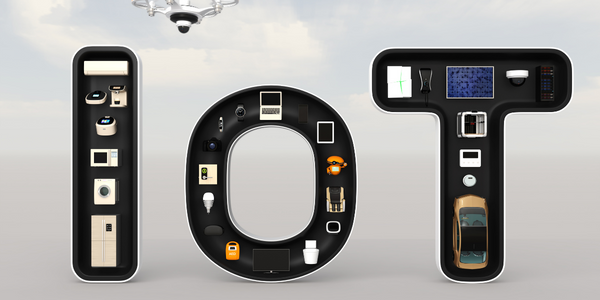
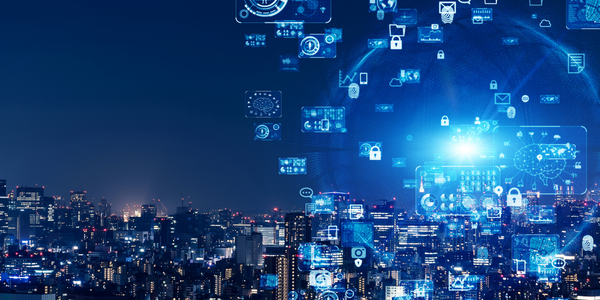
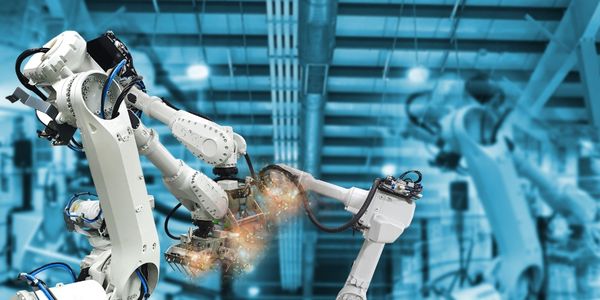