Customer Company Size
Large Corporate
Country
- United States
Product
- IBM® BigInsights®
- IBM Counter Fraud Management
Tech Stack
- Big Data Analytics
- Forensic Data Analytics
- Text Mining
Implementation Scale
- Enterprise-wide Deployment
Impact Metrics
- Cost Savings
- Productivity Improvements
Technology Category
- Analytics & Modeling - Big Data Analytics
- Application Infrastructure & Middleware - Data Exchange & Integration
Applicable Industries
- Finance & Insurance
Applicable Functions
- Business Operation
Use Cases
- Fraud Detection
- Regulatory Compliance Monitoring
Services
- Data Science Services
About The Customer
EY is a global leader in assurance, tax, transaction, and advisory services. The insights and quality services EY delivers help build trust and confidence in the capital markets and in economies the world over, and help to build a better working world for EY’s people, clients, and communities. EY provides its clients with comprehensive protection against fraud and security risks. Over the past decade, the staggering increase in volume, variety, and velocity of business information has changed how companies approach fraud and corruption investigations and compliance monitoring.
The Challenge
EY's clients are increasingly seeking growth in markets with higher perceived levels of fraud, bribery, and corruption risk. Regulators and law enforcement bodies are intensifying their activities, leading to significant corporate investment in employee training, policy development, and internal audit procedures designed to raise awareness of anti-fraud or anti-corruption policies. Many companies have also increased the use of more sophisticated, proactive uses of forensic data analytics (FDA) capabilities designed to prevent and detect areas of fraud, waste, and abuse. However, traditional rules-based tests and spreadsheet tools are not effective in managing these risks. EY needed a solution that could help them get ahead of the curve and squash potential threats before they escalate.
The Solution
EY selected IBM® Counter Fraud Management and IBM BigInsights® as the technology foundation for a first-of-its-kind offering: EY Counter Fraud. This offering has been configured exclusively to combine decades of EY’s fraud, investigation, and compliance experience and industry-specific knowledge with the big data computing power, intelligence, and scalability of IBM technology. IBM Counter Fraud Management is delivered as an all-in-one, forward-looking platform which uses comprehensive analytics to detect and highlight potentially suspect activity. Combined with the big data capabilities of IBM BigInsights, the solution allows EY to detect any potential fraud, respond promptly by applying fraud insights, investigate suspicious activity and review historical data, analyze patterns and build watch lists to monitor potentially fraudulent activities.
Operational Impact
Quantitative Benefit
Case Study missing?
Start adding your own!
Register with your work email and create a new case study profile for your business.
Related Case Studies.
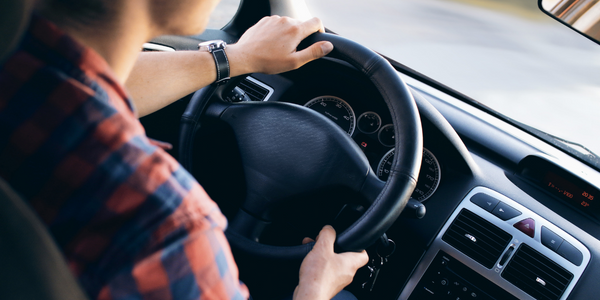
Case Study
Real-time In-vehicle Monitoring
The telematic solution provides this vital premium-adjusting information. The solution also helps detect and deter vehicle or trailer theft – as soon as a theft occurs, monitoring personnel can alert the appropriate authorities, providing an exact location.“With more and more insurance companies and major fleet operators interested in monitoring driver behaviour on the grounds of road safety, efficient logistics and costs, the market for this type of device and associated e-business services is growing rapidly within Italy and the rest of Europe,” says Franco.“The insurance companies are especially interested in the pay-per-use and pay-as-you-drive applications while other organisations employ the technology for road user charging.”“One million vehicles in Italy currently carry such devices and forecasts indicate that the European market will increase tenfold by 2014.However, for our technology to work effectively, we needed a highly reliable wireless data network to carry the information between the vehicles and monitoring stations.”
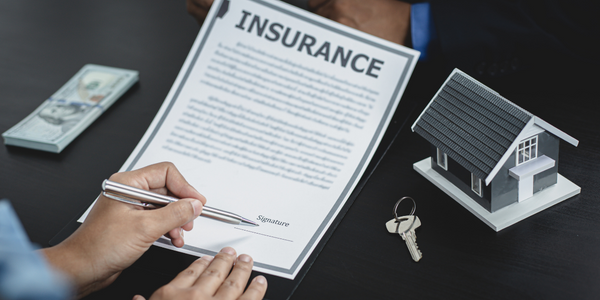
Case Study
Safety First with Folksam
The competitiveness of the car insurance market is driving UBI growth as a means for insurance companies to differentiate their customer propositions as well as improving operational efficiency. An insurance model - usage-based insurance ("UBI") - offers possibilities for insurers to do more efficient market segmentation and accurate risk assessment and pricing. Insurers require an IoT solution for the purpose of data collection and performance analysis
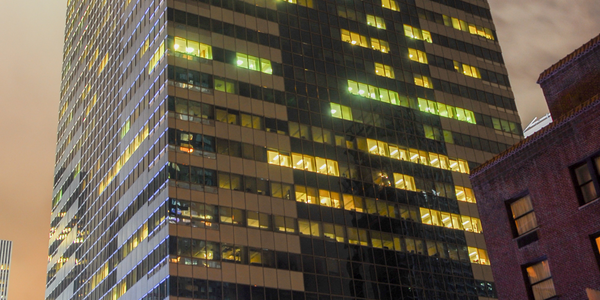
Case Study
Smooth Transition to Energy Savings
The building was equipped with four end-of-life Trane water cooled chillers, located in the basement. Johnson Controls installed four York water cooled centrifugal chillers with unit mounted variable speed drives and a total installed cooling capacity of 6,8 MW. Each chiller has a capacity of 1,6 MW (variable to 1.9MW depending upon condenser water temperatures). Johnson Controls needed to design the equipment in such way that it would fit the dimensional constraints of the existing plant area and plant access route but also the specific performance requirements of the client. Morgan Stanley required the chiller plant to match the building load profile, turn down to match the low load requirement when needed and provide an improvement in the Energy Efficiency Ratio across the entire operating range. Other requirements were a reduction in the chiller noise level to improve the working environment in the plant room and a wide operating envelope coupled with intelligent controls to allow possible variation in both flow rate and temperature. The latter was needed to leverage increased capacity from a reduced number of machines during the different installation phases and allow future enhancement to a variable primary flow system.
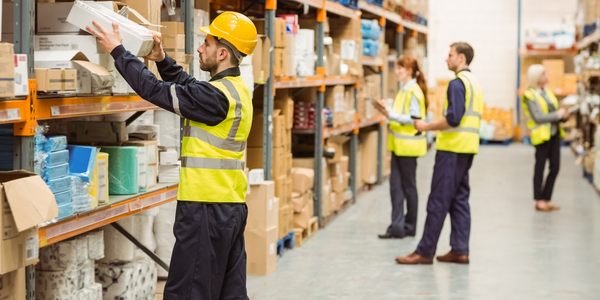
Case Study
Automated Pallet Labeling Solution for SPR Packaging
SPR Packaging, an American supplier of packaging solutions, was in search of an automated pallet labeling solution that could meet their immediate and future needs. They aimed to equip their lines with automatic printer applicators, but also required a solution that could interface with their accounting software. The challenge was to find a system that could read a 2D code on pallets at the stretch wrapper, track the pallet, and flag any pallets with unread barcodes for inspection. The pallets could be single or double stacked, and the system needed to be able to differentiate between the two. SPR Packaging sought a system integrator with extensive experience in advanced printing and tracking solutions to provide a complete traceability system.
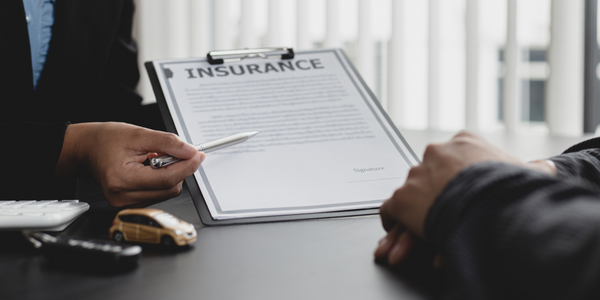
Case Study
Transforming insurance pricing while improving driver safety
The Internet of Things (IoT) is revolutionizing the car insurance industry on a scale not seen since the introduction of the car itself. For decades, premiums have been calculated using proxy-based risk assessment models and historical data. Today, a growing number of innovative companies such as Quebec-based Industrielle Alliance are moving to usage-based insurance (UBI) models, driven by the advancement of telematics technologies and smart tracking devices.
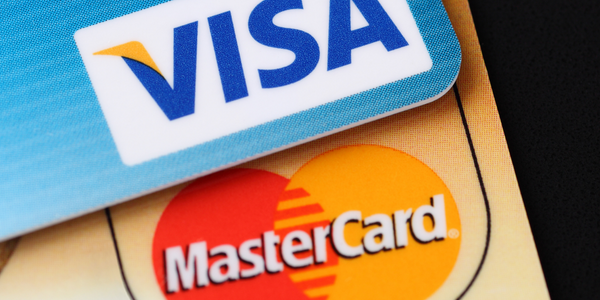
Case Study
MasterCard Improves Customer Experience Through Self-Service Data Prep
Derek Madison, Leader of Business Financial Support at MasterCard, oversees the validation of transactions and cash between two systems, whether they’re MasterCard owned or not. He was charged with identifying new ways to increase efficiency and improve MasterCard processes. At the outset, the 13-person team had to manually reconcile system interfaces using reports that resided on the company’s mainframe. Their first order of business each day was to print 20-30 individual, multi-page reports. Using a ruler to keep their place within each report, they would then hand-key the relevant data, line by line, into Excel for validation. “We’re talking about a task that took 40-80 hours each week,” recalls Madison, “As a growing company with rapidly expanding product offerings, we had to find a better way to prepare this data for analysis.”