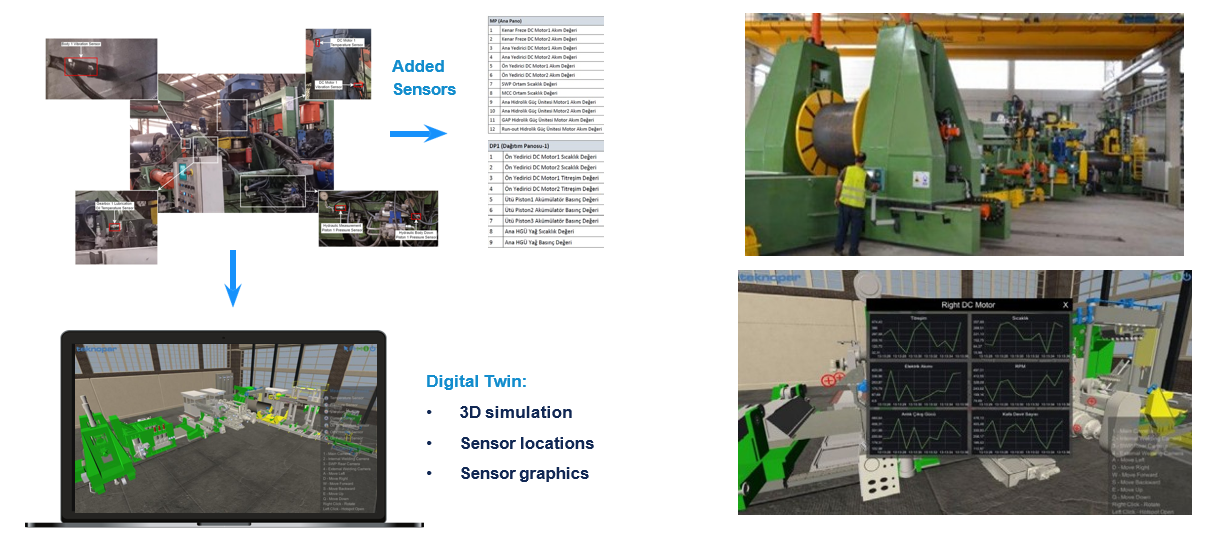
- Analytics & Modeling - Digital Twin / Simulation
- Analytics & Modeling - Machine Learning
- Analytics & Modeling - Predictive Analytics
- Analytics & Modeling - Process Analytics
- Platform as a Service (PaaS) - Data Management Platforms
- Sensors - Chemical Sensors
- Sensors - Pressure Sensors
- Sensors - Temperature Sensors
- Sensors - Voltage Sensors
- Equipment & Machinery
- Metals
- Process Manufacturing
- Digital Twin
- Machine Condition Monitoring
- Predictive Maintenance
- Software Design & Engineering Services
A Leading Spiral Welded Steel Pipe Manufacturer in Turkey
Predictive Maintenance is a sophisticated approach in equipment management that employs machine learning to constantly monitor and evaluate the condition of machinery. This methodology aids manufacturers in predicting potential faults, significantly reducing production costs, optimizing device usage, and enhancing productivity. Key activities in predictive maintenance include continuous monitoring of equipment health, data-driven condition assessment, and using advanced algorithms for predicting potential failures. A digital twin—a digital replica of a physical object, contextualized within its environment—plays a crucial role in this process.
A leading spiral welded steel pipe manufacturer in Turkey faced significant production challenges. The factory's production process, reliant on a series of interdependent machines, was highly susceptible to disruptions. Any machine failure would halt the entire production line, leading to unpredictable and prolonged downtimes. Additionally, the lack of sufficient failure data necessitated the generation of synthetic data using high-fidelity hybrid models.
TEKNOPAR implemented its TIA Platform in the factory, deploying an array of sensors on the machines to collect comprehensive data. This included 26 temperature sensors, 11 vibration sensors, 10 current sensors, 12 pressure sensors, and 1 oil contamination sensor. A cognitive digital twin was developed to provide real-time monitoring of the production process and machine status.
Upon activation, the system detected abnormally high temperatures in a machine. Initially, the operators and maintenance team doubted these readings. However, verification using a thermal camera confirmed the accuracy of the digital twin's data, leading to the immediate shutdown of the machine to prevent further damage. This quick response, facilitated by the digital twin, averted a potential disaster.
Case Study missing?
Start adding your own!
Register with your work email and create a new case study profile for your business.
Related Case Studies.
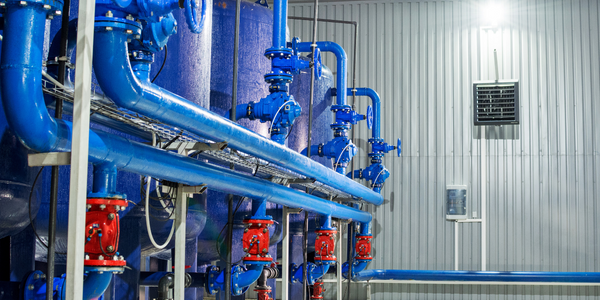
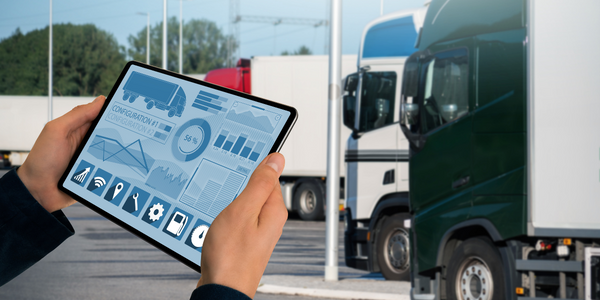
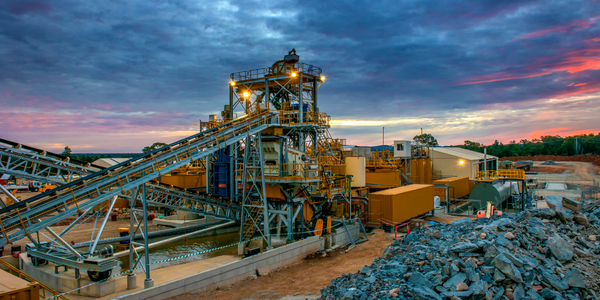
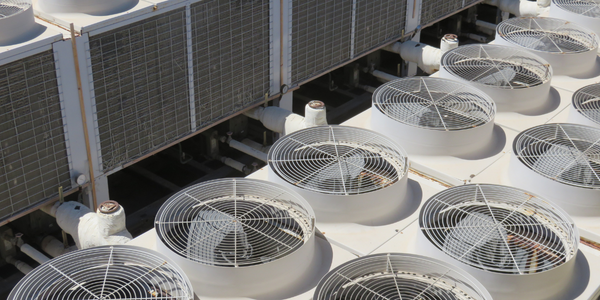
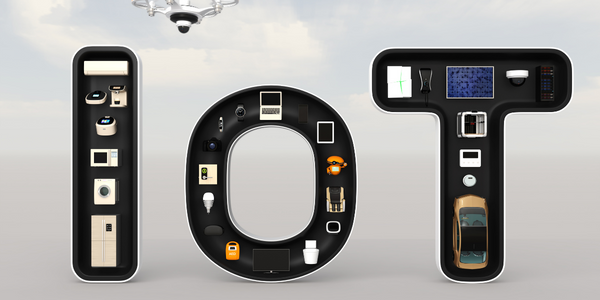
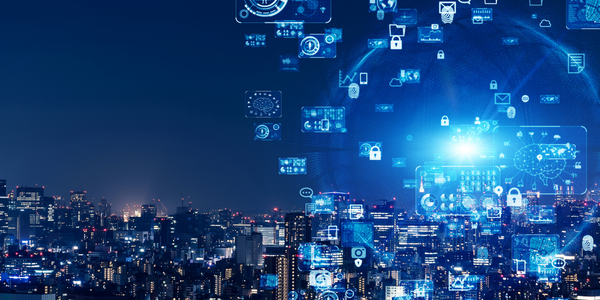