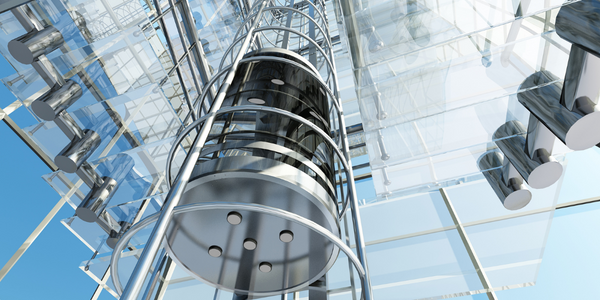
- Analytics & Modeling - Machine Learning
- Analytics & Modeling - Predictive Analytics
- Infrastructure as a Service (IaaS)
- Platform as a Service (PaaS) - Data Management Platforms
- Equipment & Machinery
- Maintenance
- Predictive Maintenance
TKE had a number of initiatives underway around the world to enable remote monitoring of its elevators. However, none of the solutions provided the data and insight required to move from a traditional reactive maintenance approach to one that is predictive and even preemptive, and all were challenged by information overload issues that limited their value. TKE wanted a solution that would enable it to anticipate and quickly resolve maintenance issues for the majority of the 1.2 million elevators it services across the globe.
CGI’s development team built a pilot, cloud-based elevator monitoring system using Microsoft’s Azure-based Intelligent System Service (ISS), Microsoft’s Azure Maching Learning, HDInsight and our own Intelligent Enterprise Framework (IEF), which facilitates the rapid deployment of IoT applications. Integrated with TKE’s elevator sensors, the system harnesses data from each sensor, processes the data using business rules defined by TKE, and generates rich data insight using predictive analytics. The resulting insight is then made available to supervisors and site technicians via two different user interfaces in the form of maintenance alerts, instructions and recommendations. Feedback from users is integrated within the system, enabling it to become more accurate over time. CGI worked with Microsoft to develop predictive data models using Microsoft’s Machine Learning Azure service. Years of historical data from elevators across North America were analysed to generate sequence mappings of alarms to identify the root causes of faults. he system was implemented for a small number of elevators run by TKE in the Seattle, Washington area in the summer of 2014. The pilot project was a success, enabling TKE to reduce elevator downtime and improve resource planning, cost forecasting and maintenance scheduling. In turn, TKE has been able to provide a more competitive offering to its customers.
Case Study missing?
Start adding your own!
Register with your work email and create a new case study profile for your business.
Related Case Studies.
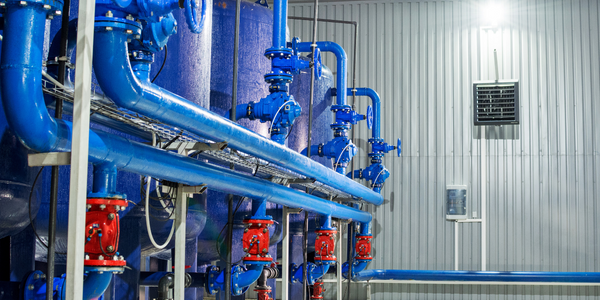
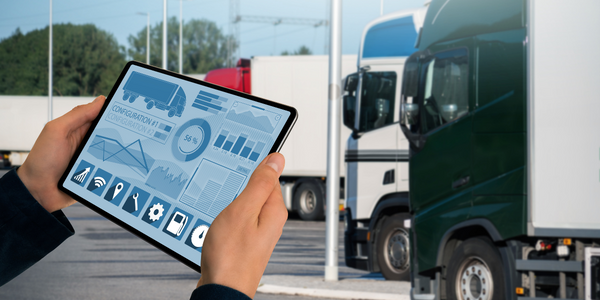
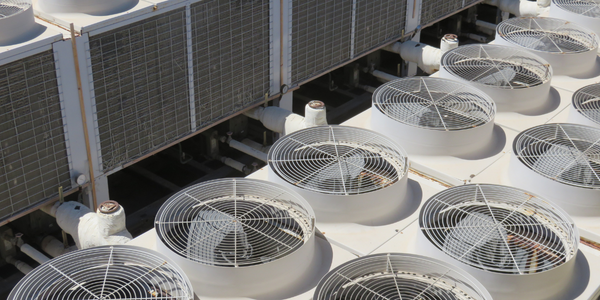
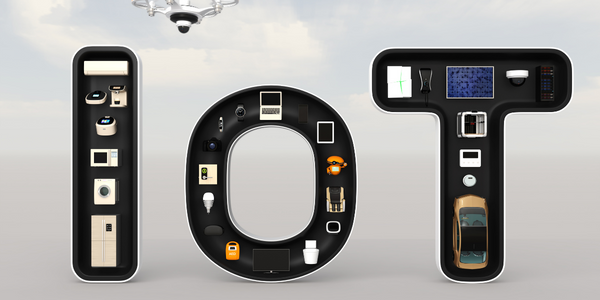
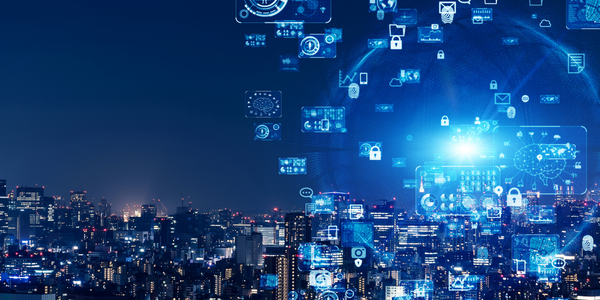
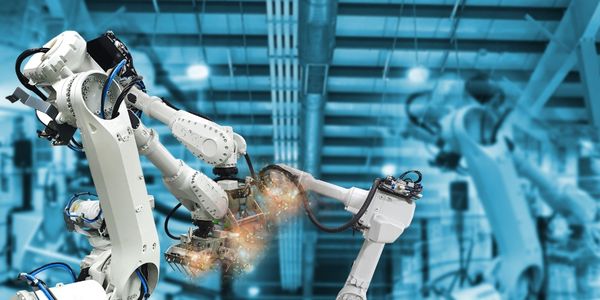