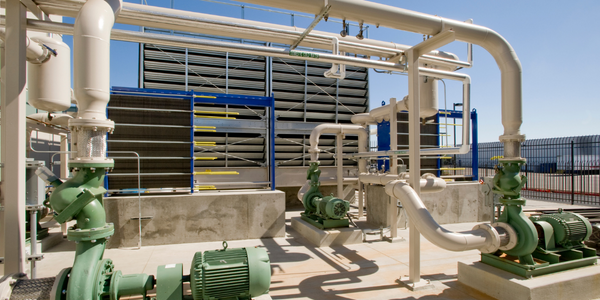
Technology Category
- Analytics & Modeling - Machine Learning
- Platform as a Service (PaaS) - Application Development Platforms
Applicable Industries
- Education
- Equipment & Machinery
Applicable Functions
- Maintenance
Use Cases
- Building Automation & Control
- Predictive Maintenance
Services
- Data Science Services
- System Integration
The Customer
Fortune 500 Manufacturing
About The Customer
The customer in this case study is the building systems division of a Fortune 500 manufacturer. This division provides equipment and services that optimize building energy expenditures, ensuring comfort for customers. The company is a global equipment manufacturer and services company with 100,000 employees and $30 billion in revenue. The company's primary objective was to load and cluster sensor data for use in a predictive model, train a machine learning model to predict chiller failure, and demonstrate the speed of development and deployment by completing the project in less than a week.
The Challenge
The building systems division of a Fortune 500 manufacturer, which provides equipment and services for optimizing building energy expenditures, was facing a significant challenge. The division was conducting chiller maintenance reactively, leading to business disruptions, downtimes, and costly emergency repairs. This reactive approach was negatively impacting customer satisfaction. The manufacturer needed a solution that could rapidly integrate all relevant equipment and facility data sources. The goal was to reduce downtime and costly, unscheduled maintenance for its commercial Heating, Venting & Cooling (HVAC) chiller systems.
The Solution
To address these challenges, the manufacturer deployed C3 AI Reliability for 165 of its chillers. The customer chose C3 AI due to its proven ability to rapidly integrate sensor data, normalize and cluster disparate readings, and run machine learning algorithms to identify deteriorating conditions before failures occur. In just 4 days, C3 AI and the customer loaded, normalized, and mapped 3 years of sensor data for all 165 chillers. They created custom analytics on these data and configured a machine learning algorithm to predict chiller failure events. C3 AI Reliability exceeded the identified accuracy and precision targets.
Operational Impact
Quantitative Benefit
Case Study missing?
Start adding your own!
Register with your work email and create a new case study profile for your business.
Related Case Studies.
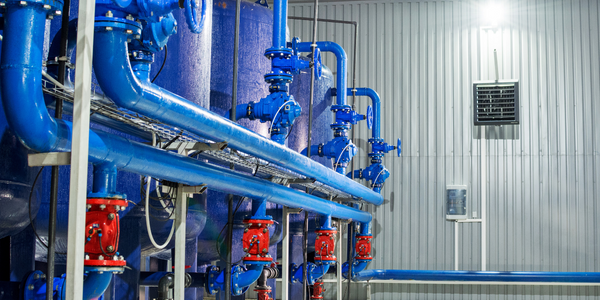
Case Study
Smart Water Filtration Systems
Before working with Ayla Networks, Ozner was already using cloud connectivity to identify and solve water-filtration system malfunctions as well as to monitor filter cartridges for replacements.But, in June 2015, Ozner executives talked with Ayla about how the company might further improve its water systems with IoT technology. They liked what they heard from Ayla, but the executives needed to be sure that Ayla’s Agile IoT Platform provided the security and reliability Ozner required.
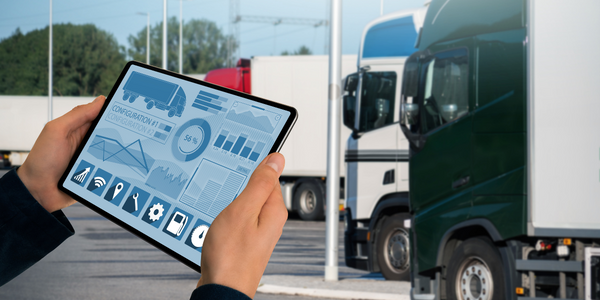
Case Study
IoT enabled Fleet Management with MindSphere
In view of growing competition, Gämmerler had a strong need to remain competitive via process optimization, reliability and gentle handling of printed products, even at highest press speeds. In addition, a digitalization initiative also included developing a key differentiation via data-driven services offers.
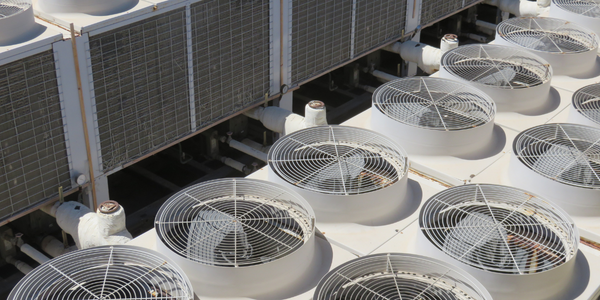
Case Study
Predictive Maintenance for Industrial Chillers
For global leaders in the industrial chiller manufacturing, reliability of the entire production process is of the utmost importance. Chillers are refrigeration systems that produce ice water to provide cooling for a process or industrial application. One of those leaders sought a way to respond to asset performance issues, even before they occur. The intelligence to guarantee maximum reliability of cooling devices is embedded (pre-alarming). A pre-alarming phase means that the cooling device still works, but symptoms may appear, telling manufacturers that a failure is likely to occur in the near future. Chillers who are not internet connected at that moment, provide little insight in this pre-alarming phase.
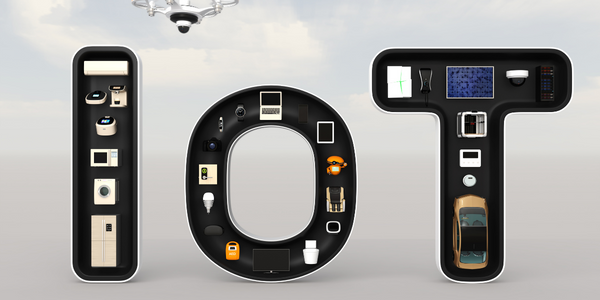
Case Study
Premium Appliance Producer Innovates with Internet of Everything
Sub-Zero faced the largest product launch in the company’s history:It wanted to launch 60 new products as scheduled while simultaneously opening a new “greenfield” production facility, yet still adhering to stringent quality requirements and manage issues from new supply-chain partners. A the same time, it wanted to increase staff productivity time and collaboration while reducing travel and costs.
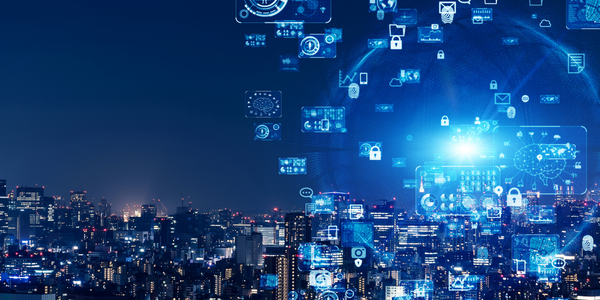
Case Study
Integration of PLC with IoT for Bosch Rexroth
The application arises from the need to monitor and anticipate the problems of one or more machines managed by a PLC. These problems, often resulting from the accumulation over time of small discrepancies, require, when they occur, ex post technical operations maintenance.
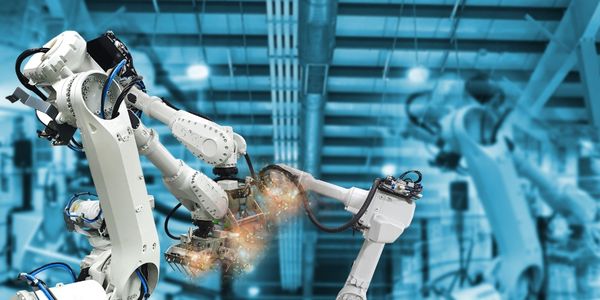
Case Study
Robot Saves Money and Time for US Custom Molding Company
Injection Technology (Itech) is a custom molder for a variety of clients that require precision plastic parts for such products as electric meter covers, dental appliance cases and spools. With 95 employees operating 23 molding machines in a 30,000 square foot plant, Itech wanted to reduce man hours and increase efficiency.