Customer Company Size
Large Corporate
Country
- Worldwide
Product
- Dataiku Data Science Studio (DSS)
Tech Stack
- Machine Learning
- Artificial Intelligence
- Internet of Things (IoT)
Implementation Scale
- Enterprise-wide Deployment
Impact Metrics
- Productivity Improvements
- Cost Savings
Technology Category
- Analytics & Modeling - Machine Learning
- Analytics & Modeling - Predictive Analytics
Applicable Functions
- Maintenance
- Discrete Manufacturing
Use Cases
- Predictive Maintenance
- Machine Condition Monitoring
Services
- Data Science Services
About The Customer
Essilor International is the world’s leading ophthalmic optics company. The company designs, manufactures, and markets a wide range of lenses to improve and protect eyesight. Essilor employs 67,000 people worldwide and has 34 plants, 481 prescription laboratories and edging facilities, as well as four research and development centers around the world. The company's core business is the production of ophthalmic lenses. In their dedication to ensure factories are efficient, innovative, and respect high-quality standards, Essilor has a Global Engineering (GE) service that is responsible for the implementation and standardization of production processes.
The Challenge
Essilor International, a leading ophthalmic optics company, was facing the challenge of improving the processes and performance of their surfacing machines to significantly enhance their production. The surfacing step in lens creation is complex and delicate, as it gives the lens its optical function. The company aimed to optimize this step to correspond to each person’s individual prescription and personal parameters. However, they were dealing with large, heterogeneous datasets from the surfacing machines and needed a scalable way to work with this data. The company was already using continuous monitoring technologies like IoT connected devices, but they wanted to take a step further by employing advanced algorithms and machine learning to take action from real-time insights.
The Solution
Essilor chose Dataiku Data Science Studio (DSS) to help them effectively work with the extensive amount of data from the surfacing machines. The setup and implementation of Dataiku were easy and allowed them to get started quickly. The tool allowed them to explore, analyze, and create predictive models that could be used by everyone, from professional experts to machine operators, data scientists, and IT. Dataiku also allowed them to manage the variations in the data from the surfacing machines efficiently and effectively. The team was able to quickly test and iterate on use cases to arrive at a solution faster. They also appreciated the flexibility to work using code or using the point-and-click visual interface, whichever allowed them to work more quickly.
Operational Impact
Quantitative Benefit
Case Study missing?
Start adding your own!
Register with your work email and create a new case study profile for your business.
Related Case Studies.
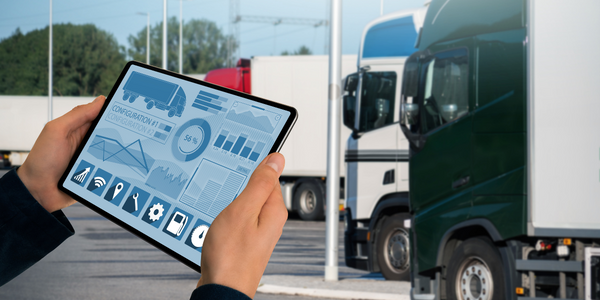
Case Study
IoT enabled Fleet Management with MindSphere
In view of growing competition, Gämmerler had a strong need to remain competitive via process optimization, reliability and gentle handling of printed products, even at highest press speeds. In addition, a digitalization initiative also included developing a key differentiation via data-driven services offers.
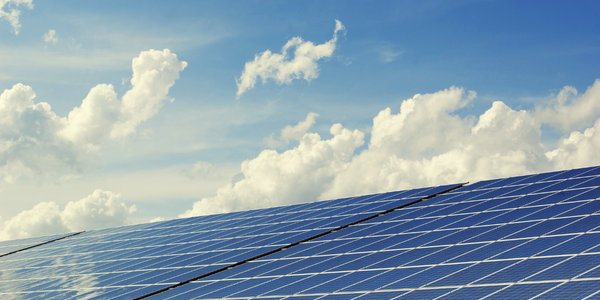
Case Study
Remote Monitoring & Predictive Maintenance App for a Solar Energy System
The maintenance & tracking of various modules was an overhead for the customer due to the huge labor costs involved. Being an advanced solar solutions provider, they wanted to ensure early detection of issues and provide the best-in-class customer experience. Hence they wanted to automate the whole process.
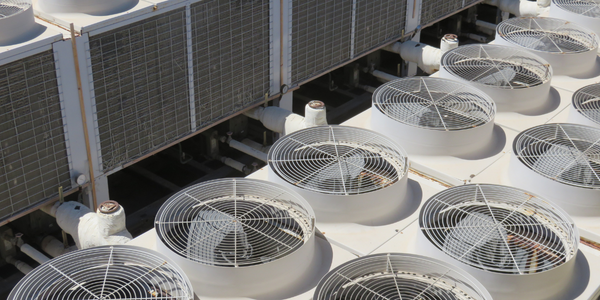
Case Study
Predictive Maintenance for Industrial Chillers
For global leaders in the industrial chiller manufacturing, reliability of the entire production process is of the utmost importance. Chillers are refrigeration systems that produce ice water to provide cooling for a process or industrial application. One of those leaders sought a way to respond to asset performance issues, even before they occur. The intelligence to guarantee maximum reliability of cooling devices is embedded (pre-alarming). A pre-alarming phase means that the cooling device still works, but symptoms may appear, telling manufacturers that a failure is likely to occur in the near future. Chillers who are not internet connected at that moment, provide little insight in this pre-alarming phase.
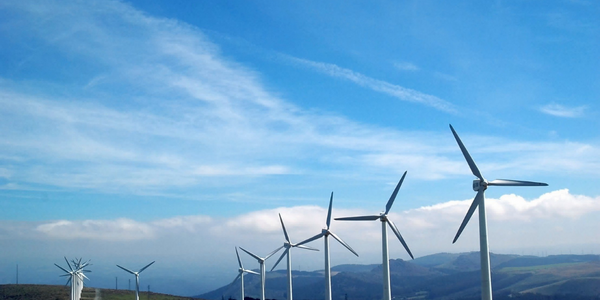
Case Study
Siemens Wind Power
Wind provides clean, renewable energy. The core concept is simple: wind turbines spin blades to generate power. However, today's systems are anything but simple. Modern wind turbines have blades that sweep a 120 meter circle, cost more than 1 million dollars and generate multiple megawatts of power. Each turbine may include up to 1,000 sensors and actuators – integrating strain gages, bearing monitors and power conditioning technology. The turbine can control blade speed and power generation by altering the blade pitch and power extraction. Controlling the turbine is a sophisticated job requiring many cooperating processors closing high-speed loops and implementing intelligent monitoring and optimization algorithms. But the real challenge is integrating these turbines so that they work together. A wind farm may include hundreds of turbines. They are often installed in difficult-to-access locations at sea. The farm must implement a fundamentally and truly distributed control system. Like all power systems, the goal of the farm is to match generation to load. A farm with hundreds of turbines must optimize that load by balancing the loading and generation across a wide geography. Wind, of course, is dynamic. Almost every picture of a wind farm shows a calm sea and a setting sun. But things get challenging when a storm goes through the wind farm. In a storm, the control system must decide how to take energy out of gusts to generate constant power. It must intelligently balance load across many turbines. And a critical consideration is the loading and potential damage to a half-billion-dollar installed asset. This is no environment for a slow or undependable control system. Reliability and performance are crucial.
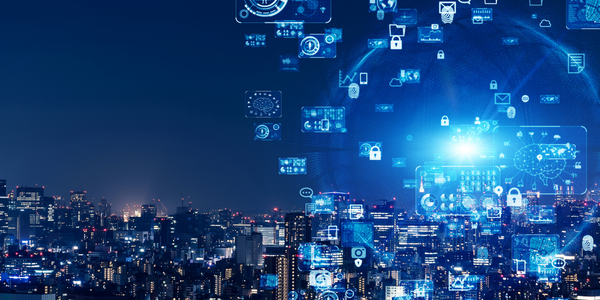
Case Study
Integration of PLC with IoT for Bosch Rexroth
The application arises from the need to monitor and anticipate the problems of one or more machines managed by a PLC. These problems, often resulting from the accumulation over time of small discrepancies, require, when they occur, ex post technical operations maintenance.
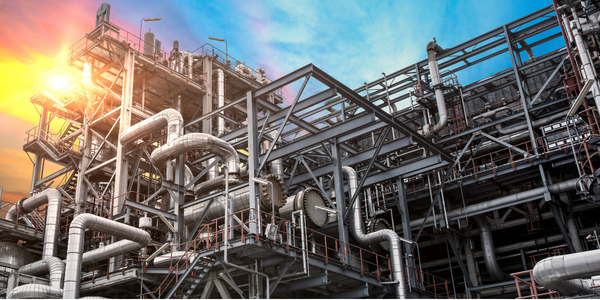
Case Study
Refinery Saves Over $700,000 with Smart Wireless
One of the largest petroleum refineries in the world is equipped to refine various types of crude oil and manufacture various grades of fuel from motor gasoline to Aviation Turbine Fuel. Due to wear and tear, eight hydrogen valves in each refinery were leaking, and each cost $1800 per ton of hydrogen vented. The plant also had leakage on nearly 30 flare control hydrocarbon valves. The refinery wanted a continuous, online monitoring system that could catch leaks early, minimize hydrogen and hydrocarbon production losses, and improve safety for maintenance.