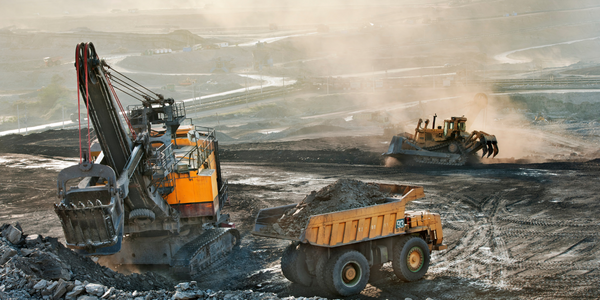
- Analytics & Modeling - Machine Learning
- Analytics & Modeling - Predictive Analytics
- Infrastructure as a Service (IaaS)
- Mining
- Maintenance
- Predictive Maintenance
Mining companies have a lot of data at their disposal. Sensors are seemingly everywhere in their underground operations. But thus far it has been very hard for mining companies to capitalize on all their data because of the difficulty in making sense of it all.
So what’s the most important data for mining companies? The short answer: assets. Mining is one of the most asset-intensive businesses there is. At every point in the extraction chain— drilling, cutting, crushing, screening and removing ore-bearing rock—heavy equipment is critical. And it takes a beating. When equipment breaks down, requiring unscheduled maintenance, production takes a hit, costs rise and a critical measure of capital efficiency in mining—overall equipment effectiveness (OEE)—goes down.
IBM uses machine learning algorithms to analyze the equipment sensor data at a component level. The idea is as basic as it is powerful: if you analyze a large enough dataset on the maintenance and failure pattern for a particular component, you’ll be able to make an accurate prediction of when that component—say, part of an engine, a transmission or brakes—is likely to fail. The central insight these models produce—a prediction of each component’s lifetime—is tremendously powerful because it gives operators the critical element they need to optimize scheduled maintenance practices across their entire operations for all their equipment.
Case Study missing?
Start adding your own!
Register with your work email and create a new case study profile for your business.
Related Case Studies.
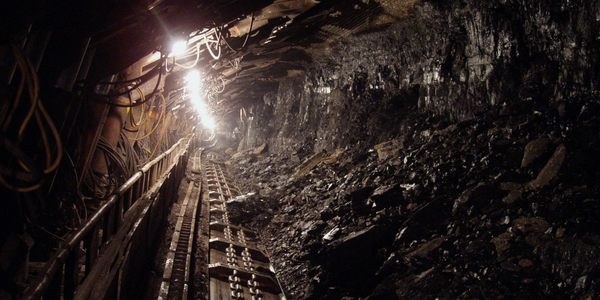
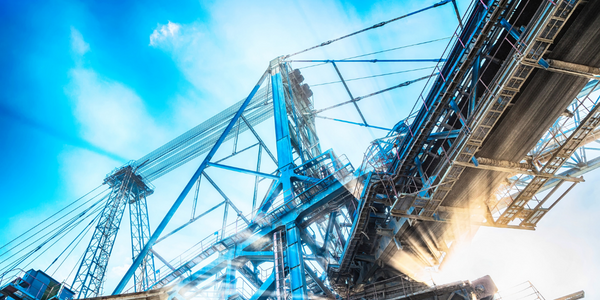
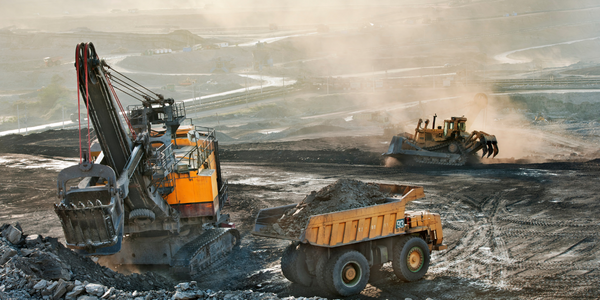
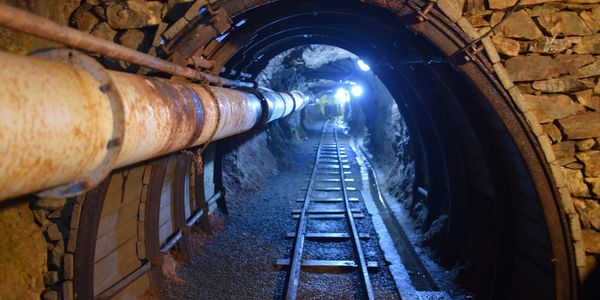
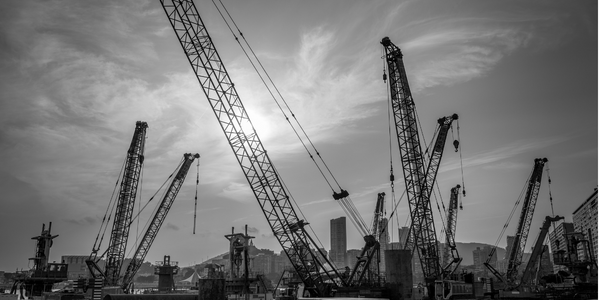