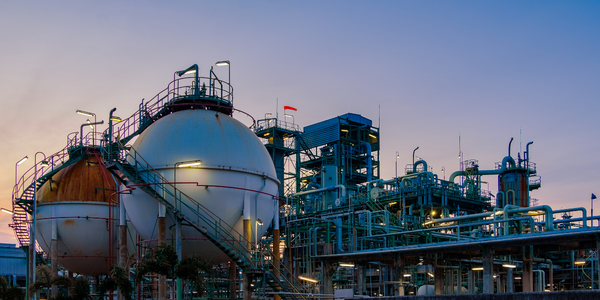
- Analytics & Modeling - Predictive Analytics
- Automation & Control - Supervisory Control & Data Acquisition (SCADA)
- Functional Applications - Computerized Maintenance Management Systems (CMMS)
- Sensors - Temperature Sensors
- Sensors - Vibration Sensors
- Sensors - Voltage Sensors
- Oil & Gas
- Maintenance
- Predictive Maintenance
The challenge was to harvest and sift through this data, recognize the patterns that indicate a high likelihood of asset failure, identify the most urgent issues, and get the right information to its engineers with enough lead time for them to take effective action.
“Before, we only used between 10 and 12 percent of the operational data we collected, which is the industry average,” comments Benn. “By the time we had searched for, collated and forwarded the right information to the right people, we might respond too late to avoid impact to operations, or have to make last-minute changes to our maintenance schedule, which reduces efficiency. Our challenge was to provide right-time, actionable, effective information proactively, rather than in a reactive or look-back assessment.”
“What we wanted was a way to identify patterns in that sensor data that would give us an early warning of asset failure. We saw an opportunity to use analytics technology to extract greater value from the systems and data we already possessed, which would help us to, for example, avoid preventable failures and potentially save millions of dollars.
To create an effective alert system for equipment failure, Santos looked to predictive modelling. Embarking on a pilot project with help from IBM® SPSS® Lab Services, the company pulled structured and unstructured data from multiple sources including: the equipment trends database, which tracks SCADA data such as the speed at which a compressor is operating; the operator shift log, a system in which field operatives record their activity; the computerized maintenance management system, which maintains a record of assets and their maintenance history; and the asset loss and availability system, an accounting solution used to trace the sources of production losses.
Steven Benn says: “We can now identify issues from overall pattern-matching and analysis across all relevant data streams using signals from multiple sources. It is this holistic picture that we analyze to generate warnings and notifications for our engineers and field operators. The majority of these warnings cannot be derived from a single source or point sensors, and so would otherwise remain undetected.”
Case Study missing?
Start adding your own!
Register with your work email and create a new case study profile for your business.
Related Case Studies.
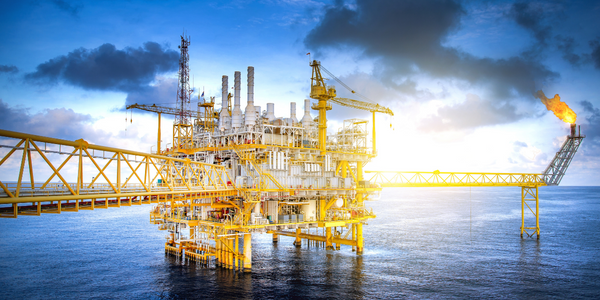
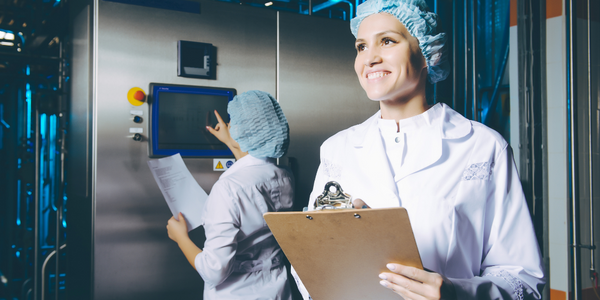
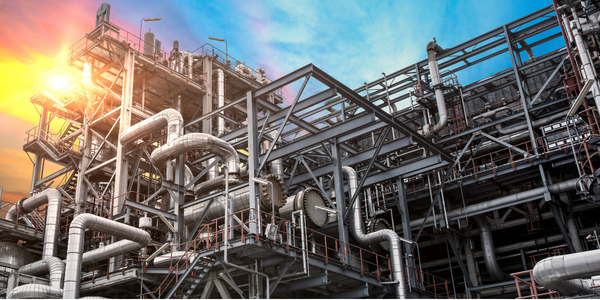