Clinical Image Analysis
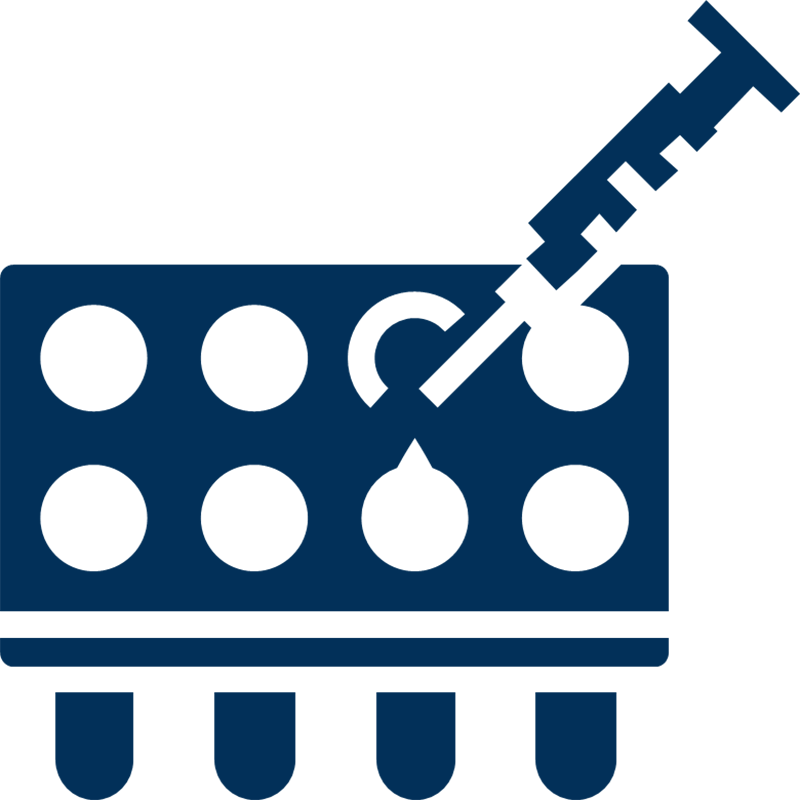
Clinical image analysis is the technique and process of creating visual representations of the interior of a body for clinical analysis and medical intervention. Clinicians are able to better visualize and interpret the situation inside bodies. Artificial Intelligence solutions can use image data to provide faster and more accurate diagnosis and treatment. According to research done at Harvard Medical School, a patient’s hospital stay is reduced by one day for every $385 spent on medical imaging equipment in the United States. The demand for cheaper, portable, and miniature versions of clinical image analysis tools is rising, especially in developing economies due to a shortage of trained physicians.
Improved Diagnostic Accuracy: Clinical image analysis enables healthcare providers to achieve higher levels of diagnostic accuracy and precision by leveraging advanced algorithms and machine learning techniques. By assisting radiologists and clinicians in the interpretation of medical images, clinical image analysis helps identify subtle abnormalities, lesions, or patterns that may be overlooked or misinterpreted by the human eye alone, leading to more accurate diagnoses and treatment decisions.
Enhanced Efficiency and Productivity: By automating routine tasks such as image segmentation, feature extraction, and quantitative analysis, clinical image analysis streamlines workflows and enhances operational efficiency in healthcare facilities. Automated analysis reduces the time and effort required for manual interpretation, enabling radiologists and clinicians to focus their expertise on complex cases and critical decision-making tasks, thereby increasing productivity and throughput.
Healthcare Providers: Healthcare providers, including radiologists, clinicians, and medical imaging specialists, are primary stakeholders in clinical image analysis. They rely on image analysis tools and software to interpret medical images, make diagnostic decisions, and develop treatment plans for patients. Healthcare providers contribute domain expertise, clinical insights, and feedback to inform the development and refinement of image analysis algorithms and applications.
Patients: Patients are essential stakeholders whose health outcomes and well-being are impacted by clinical image analysis. Patients benefit from accurate diagnosis, personalized treatment, and timely interventions facilitated by image analysis technology. Patient stakeholders expect transparency, informed consent, and privacy protection in the use of their medical imaging data for analysis and diagnosis, emphasizing the importance of ethical considerations and patient-centered care in image analysis practices.
Regulatory Agencies and Policymakers: Regulatory agencies and policymakers play a critical role in setting standards, regulations, and guidelines governing the development, validation, and deployment of clinical image analysis solutions. Regulatory stakeholders ensure that image analysis products comply with quality standards, safety requirements, and ethical principles, protecting patient safety and promoting the responsible use of technology in healthcare.
Image Acquisition and Processing: Technology facilitates image acquisition from various modalities such as X-ray, MRI, CT scans, ultrasound, and PET scans. Advanced imaging technologies, such as 3D imaging and spectral imaging, provide rich datasets for analysis. Image processing techniques, including filtering, enhancement, and registration, are employed to preprocess raw images and improve their quality for subsequent analysis.
Machine Learning and Artificial Intelligence: Machine learning (ML) and artificial intelligence (AI) algorithms are at the forefront of clinical image analysis, enabling automated interpretation, segmentation, and classification of medical images. Deep learning architectures, such as convolutional neural networks (CNNs) and recurrent neural networks (RNNs), are widely used for feature extraction, pattern recognition, and predictive modeling tasks, leading to advancements in computer-aided diagnosis, lesion detection, and disease staging.
Data Acquisition and Preprocessing: Data acquisition involves collecting medical imaging data from various modalities, such as X-rays, MRI, CT scans, and ultrasound. Preprocessing techniques, including noise reduction, image normalization, and artifact correction, are applied to enhance the quality and consistency of imaging data, ensuring optimal performance of image analysis algorithms.
Annotation and Labeling: Annotation and labeling processes involve manually or semi-automatically annotating medical images with regions of interest, anatomical landmarks, or pathological findings. Annotated data serves as ground truth for training and validating machine learning models, enabling supervised learning approaches for image analysis tasks such as segmentation, classification, and detection.
Data Privacy and Security: Data privacy and security measures are essential to protect patient confidentiality and comply with regulatory requirements, such as HIPAA (Health Insurance Portability and Accountability Act) in the United States and GDPR (General Data Protection Regulation) in the European Union. Healthcare organizations must implement encryption, access controls, and audit trails to safeguard sensitive medical imaging data from unauthorized access, disclosure, or misuse.
Infrastructure and Hardware Requirements: Deployment of clinical image analysis solutions requires robust infrastructure and hardware resources capable of processing and storing large volumes of imaging data. High-performance computing (HPC) clusters, cloud-based infrastructure, or specialized hardware accelerators, such as graphics processing units (GPUs) or tensor processing units (TPUs), may be employed to support computationally intensive tasks, such as deep learning-based image analysis algorithms.
Integration with Clinical Workflows: Integration with existing clinical workflows and electronic health record (EHR) systems is essential to ensure seamless adoption and interoperability of image analysis solutions in healthcare settings. Deployed solutions should integrate with radiology information systems (RIS), picture archiving and communication systems (PACS), and other clinical systems to streamline image acquisition, analysis, reporting, and decision support processes.
User Training and Support: User training and support programs are necessary to familiarize healthcare professionals with the use of image analysis software, interpret algorithm outputs, and incorporate AI-driven insights into clinical decision-making. Training sessions, educational resources, and technical support services help users navigate the deployment challenges, optimize workflow efficiency, and maximize the value of image analysis solutions in patient care.