Predictive Quality Analytics
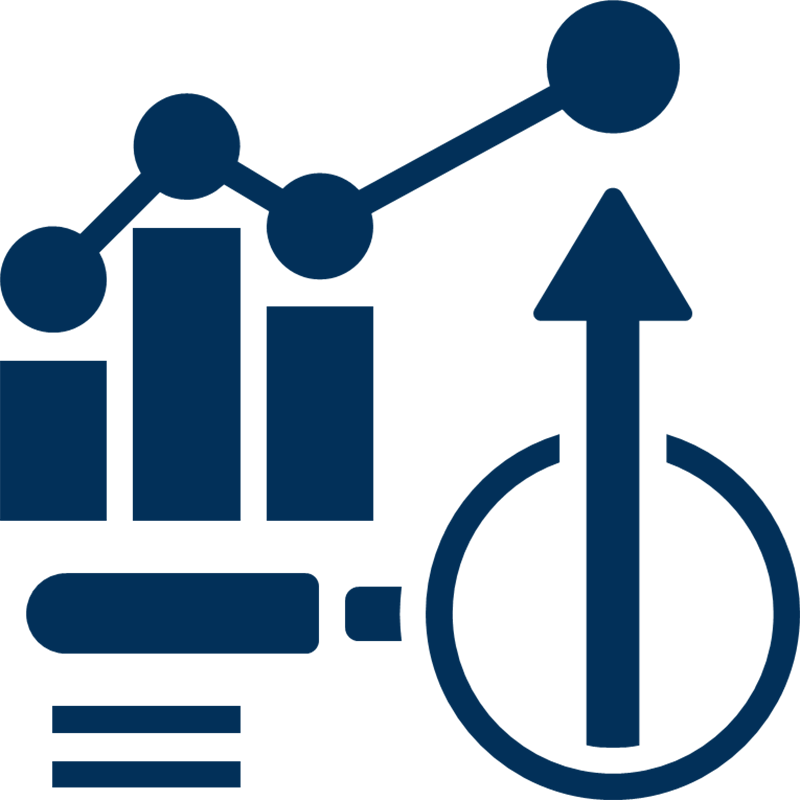
Overview
Predictive quality analytics uses statistical algorithms and Machine Learnings to anticipate quality and safety risks before they occur, offering the opportunity to take timely and targeted countermeasures. As a first step, all available external and internal data sources are prioritized, consolidated, and correlated. Comprehensive data analyses are then performed and predictive models are developed in an iterative process, making use of a variety of evaluation techniques. Depending on the parameters which flow into the model, it is possible with these methods to forecast not only defects that appear shortly in the future but also ones which could lead to a warranty claim in the long term. The models are thus capable of integrating data sources, making efficient Data Mining possible and leading to user-friendly evaluations which in turn can be converted into easily readable reports for the end-user or managers at the reporting level. Wisely used, predictive quality analytics can lead to significant savings in warranty costs, improve customer satisfaction, and reduce scrap rates.