公司规模
Large Corporate
国家
- Worldwide
产品
- SendinBlue
- Dataiku Data Science Studio (DSS)
技术栈
- MongoDB
- MySQL
- Redshift
- Python
实施规模
- Enterprise-wide Deployment
影响指标
- Productivity Improvements
- Customer Satisfaction
技术
- 分析与建模 - 预测分析
适用功能
- 销售与市场营销
用例
- 欺诈识别
- 质量预测分析
服务
- 数据科学服务
关于客户
SendinBlue 是一款关系营销 SaaS 解决方案,于 2012 年推出。该公司的使命是成为最简单、最可靠、最具成本效益的营销平台。SendinBlue 目前为全球 50,000 多家公司提供营销活动支持。SendinBlue 团队在北美、欧洲和亚洲设有办事处,支持六种语言的产品。他们的平台与顶级电子商务和 CMS 工具集成,他们的系统每天发送超过 3000 万封电子邮件和短信。
挑战
SendinBlue 是一家关系营销 SaaS 解决方案公司,在验证新客户和确保数据库质量方面面临巨大挑战。该公司必须确保列表中的所有联系人都已选择加入,这需要手动验证。这个过程不仅耗时且需要大量人力,而且还严重延迟了客户的帐户验证,损害了 SendinBlue 的声誉。随着客户群的增长,手动验证变得越来越不可行。该公司需要一种可以自动化验证过程并随着需求增长而扩展的解决方案。
解决方案
SendinBlue 借助 Dataiku 数据科学工作室 (DSS) 开发了一套自动欺诈检测系统。SendinBlue 使用来自超过 10 亿封电子邮件和相关事件的历史数据、数千个被阻止的账户和数百个欺诈标准,构建了一个可扩展的解决方案。新系统会分析新客户,并自动将其归类为“好”、“坏”或“不确定”。然后,算法会通过考虑发送量、联系人的评分等来确定客户的可信度。根据客户的风险评分,他们可能会被阻止、验证或发送给客户服务部门进行人工分析。Dataiku 在将数据产品部署到生产中、处理大量不同的数据集以及在不到三个月的时间内设计、测试和开发解决方案方面发挥了重要作用。
运营影响
数量效益
Case Study missing?
Start adding your own!
Register with your work email and create a new case study profile for your business.
相关案例.
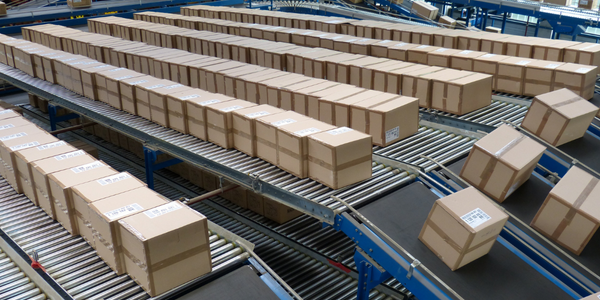
Case Study
IoT Data Analytics Case Study - Packaging Films Manufacturer
The company manufactures packaging films on made to order or configure to order basis. Every order has a different set of requirements from the product characteristics perspective and hence requires machine’s settings to be adjusted accordingly. If the film quality does not meet the required standards, the degraded quality impacts customer delivery causes customer dissatisfaction and results in lower margins. The biggest challenge was to identify the real root cause and devise a remedy for that.
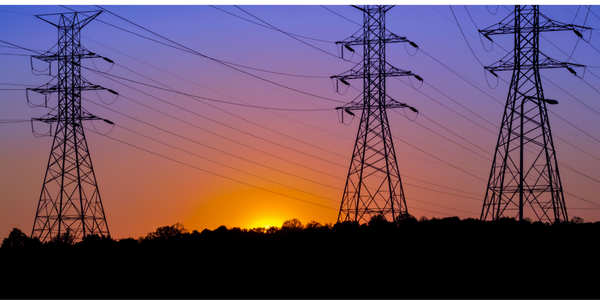
Case Study
Largest Production Deployment of AI and IoT Applications
To increase efficiency, develop new services, and spread a digital culture across the organization, Enel is executing an enterprise-wide digitalization strategy. Central to achieving the Fortune 100 company’s goals is the large-scale deployment of the C3 AI Suite and applications. Enel operates the world’s largest enterprise IoT system with 20 million smart meters across Italy and Spain.
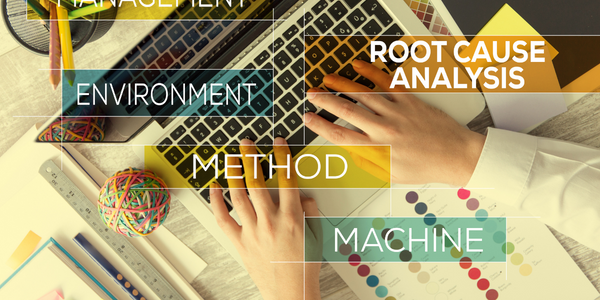
Case Study
Prevent Process Inefficiencies with Automated Root Cause Analysis
Manufacturers mostly rely on on-site expert knowledge for root cause analysis. When the defective product is sent to lab for analysis, it is laborious and always a post-mortem one. Manufacturers that collect data from IT and OT also need a comprehensive understanding of a variety of professionals to make sense of it. This is not only time consuming, but also inefficiencient.
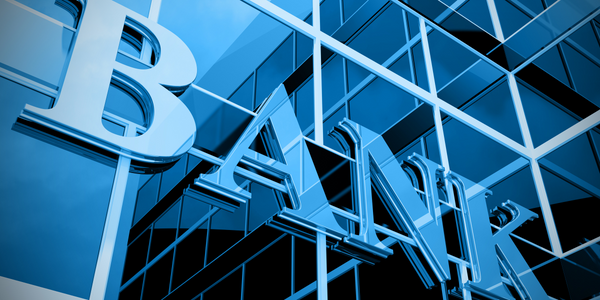
Case Study
KeyBank's Digital Transformation with Confluent's Data in Motion
KeyBank, one of the nation's largest bank-based financial services companies, embarked on a national digital bank initiative following the acquisition of Laurel Road, a digital consumer lending business. The initiative aimed to build a digital bank focused on healthcare professionals looking to refinance student loans and buy homes. A significant challenge was reducing the time to market for new products by democratizing data and decoupling systems across the IT landscape. Like many large enterprises, KeyBank had a variety of vendor applications, custom applications, and other systems that were tightly coupled to one another. New projects often required developing specific point-to-point integrations for exchanging data, which did not address the needs of other downstream systems that could benefit from the same data.
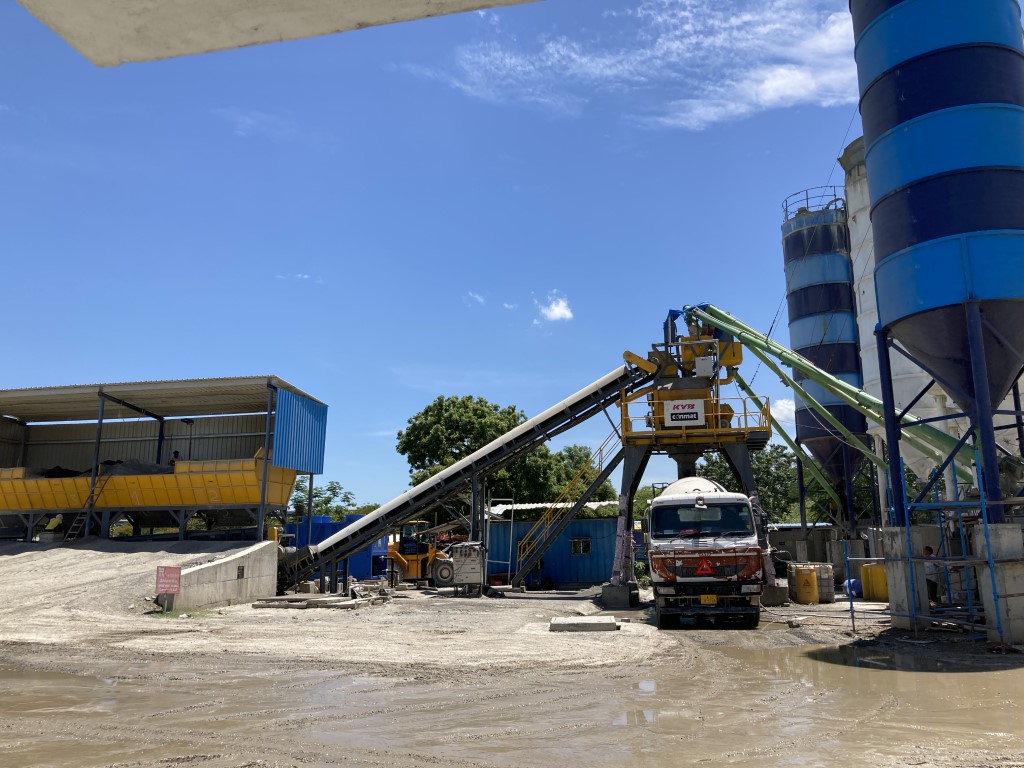
Case Study
Digitalising QC records
Ready-mix concrete batching plant with seasonal demand 6,000 to 12,000 cu.metre per month.Batch-cycle records for each truck is stored in paper format. 1000 to 2000 truck loads per month, generating ~2000 to 6000 paper records.QC anomaly detection in chemical batch-mixing is manual & time consuming.
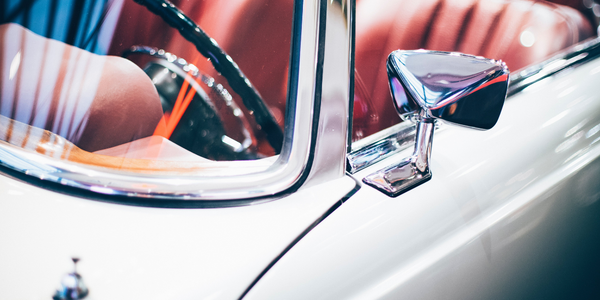
Case Study
Automotive manufacturer increases productivity for cylinder-head production by 2
Daimler AG was looking for a way to maximize the number of flawlessly produced cylinder-heads at its Stuttgart factory by making targeted process adjustments. The company also wanted to increase productivity and shorten the ramp-up phase of its complex manufacturing process.