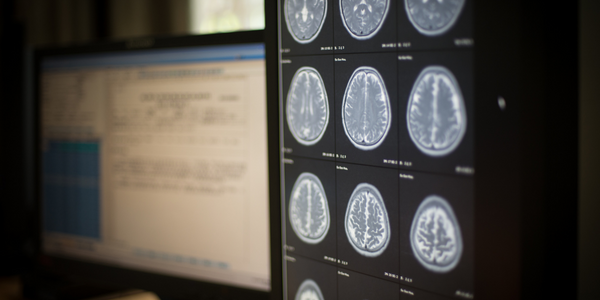
- Analytics & Modeling - Machine Learning
- Healthcare & Hospitals
- Automated Disease Diagnosis
There are several factors simultaneously driving integration of AI in radiology. Firstly, in many countries around the world there is a discrepancy between the number of doctors trained in radiology and the rising demand for diagnostic imaging. This leads to greater demands for work efficiency and productivity. For example, the number of radiology specialists (consultant work- force) in England went up 5% between 2012 and 2015, while in the same period the number of CT and MR scans increased by 29 and 26 percentage points respectively. In Scotland, the gap widened even further (The Royal College of Radiologists 2016). Today, the average radiologist is interpreting an image every three to four seconds, eight hours a day (Choi et al. 2016).
Secondly, the image resolution of today’s scanners is continuously improving – resulting in an ever greater volume of data. Indeed, the estimated overall medical data volume doubles every three years, making it harder and harder for radiologists to make good use of the available information without extra help from computerized digital processing. It is desirable, both in radiological research and in clinical diagnostics, to be able to quantitatively analyze this largely unexploited wealth of data and, for example, utilize new measurable imaging biomarkers to assess disease progression and prognosis (O’Connor et al. 2017). Experts see considerable future potential in the transformation of radiology from a discipline of qualitative interpretation to one of quantita- tive analysis, which derives clinically relevant information from extensive data sets (“radiomics”). “Images are more than pictures, they are data,” American radiologist Robert Gillies and his colleagues write (Gillies et al. 2016). Of course, this direction for radiology will require powerful, automated procedures, some of which at least will come under the field of artificial intelligence.
The use of machine learning in medical imaging is not new – algorithms today are, however, much more powerful than traditional applications (van Ginneken 2017). The ANNs on which deep learn- ing is based always have multiple functional layers, sometimes even exceeding a hundred, which can encompass thousands of neurons with millions of connections. (Simple ANNs with, say, only one interim layer are described in contrast as “shallow” networks.) All of these connections are adjusted during an ANN’s training by gradual changes of their respective parameters – in mathematical terms: their weights. In this way, deep networks feature a virtually unimaginable number of possible combinations for processing information, and can even model highly complex, non-linear contexts. During the training procedure, the different layers of an ANN increasingly structure the input data with each consecutive layer, developing a more abstract “understanding” of the information. Of course, such deep ANNs were only made possible by advanced mathe- matical methods and the availability of higher computational power and faster graphic processors (GPU) to compute the innumerable steps during the learning process. In 2013, the MIT Technology Review identified deep learning as one of the 10 Breakthrough Technologies of the Year (Hof 2013).
For image recognition, “deep convolutional neural networks” (a specific type of ANN) have proven to be especially efficient. Similar to the visual cortex in the brain, these networks first extract fundamental image characteristics from the input data, like corners, edges and shading. In multiple abstraction steps, they then settle independently on more complex image patterns and objects. When the best of these kinds of networks are tested on non-medical image databases, their error rate is now down to just a few percent (He et al. 2015). Moreover, different network architectures and methods may be combined (e.g. deep learning with “reinforcement learning”) to achieve an optimal result depending on the problem posed.
Given this development, experts anticipate significant changes in medical imaging (Lee et al. 2017). Unlike previous AI methods, introduced in the US beginning in the late 1990s especially for mammography screening with a lot of shortcomings (Morton et al. 2006; Fenton et al. 2007; Lehman et al. 2015), today’s algorithms will likely prove to be transformative technologies for clinical diagnostics.
Case Study missing?
Start adding your own!
Register with your work email and create a new case study profile for your business.
Related Case Studies.
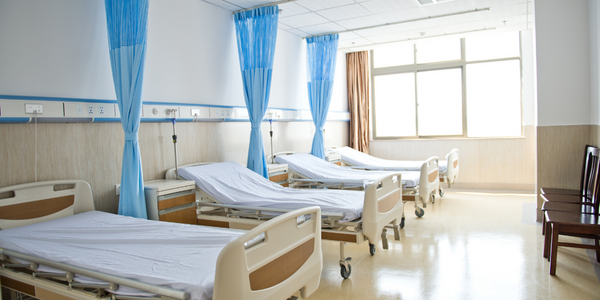
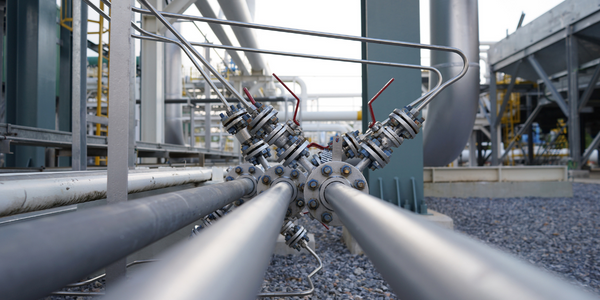
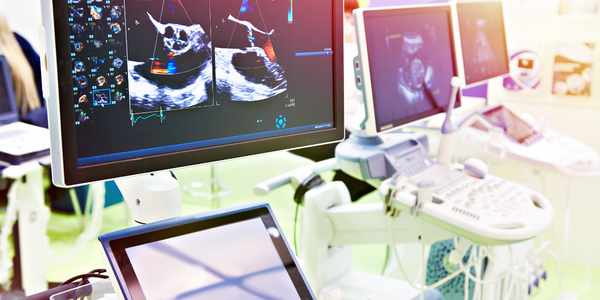
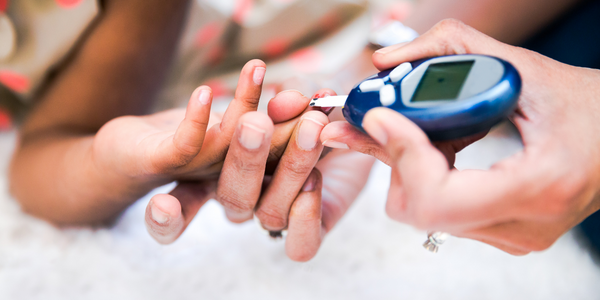
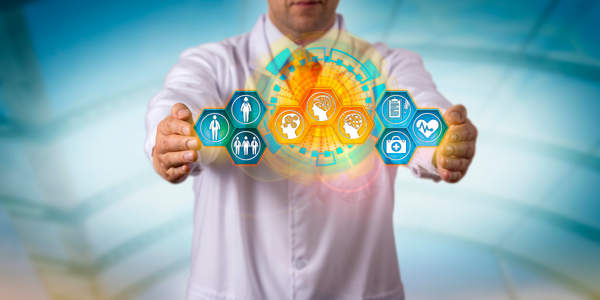