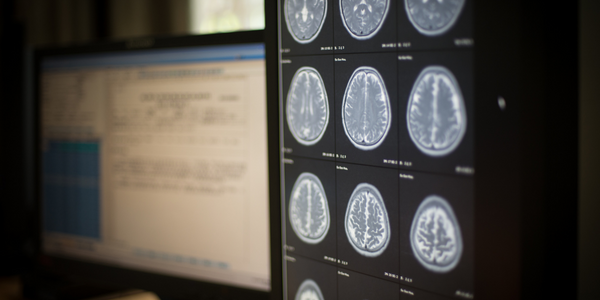
- 分析与建模 - 机器学习
- 医疗保健和医院
- 自动化疾病诊断
有几个因素同时推动人工智能在放射学中的整合。首先,在世界上许多国家,接受过放射学培训的医生数量与对诊断成像的需求不断增长之间存在差异。这导致对工作效率和生产力的更高要求。例如,2012 年至 2015 年期间,英格兰放射科专家(顾问劳动力)的数量增加了 5%,而同期 CT 和 MR 扫描的数量分别增加了 29 个和 26 个百分点。在苏格兰,差距进一步扩大(皇家放射科医师学院,2016 年)。今天,放射科医生平均每 3 到 4 秒解读一次图像,每天 8 小时(Choi 等人,2016 年)。
其次,当今扫描仪的图像分辨率不断提高——导致数据量越来越大。事实上,估计的整体医疗数据量每三年翻一番,这使得放射科医生越来越难以在没有计算机数字处理的额外帮助的情况下充分利用可用信息。在放射学研究和临床诊断中,希望能够定量分析这些大量未开发的数据,例如,利用新的可测量成像生物标志物来评估疾病进展和预后(O'Connor et al. 2017) .专家们看到放射学从定性解释学科转变为定量分析学科的巨大未来潜力,定量分析从广泛的数据集(“放射组学”)中获取临床相关信息。 “图像不仅仅是图片,它们是数据,”美国放射科医生 Robert Gillies 和他的同事写道(Gillies et al. 2016)。当然,放射学的这个方向将需要强大的自动化程序,其中一些至少将属于人工智能领域。
在医学成像中使用机器学习并不新鲜——然而,今天的算法比传统应用程序强大得多(van Ginneken 2017)。深度学习所基于的人工神经网络总是有多个功能层,有时甚至超过一百个,可以包含数千个神经元和数百万个连接。 (例如,只有一个中间层的简单人工神经网络被描述为“浅层”网络。)所有这些连接都在人工神经网络的训练过程中通过它们各自参数的逐渐变化来调整——用数学术语来说:它们的权重。通过这种方式,深度网络具有几乎无法想象的处理信息的可能组合数量,甚至可以对高度复杂的非线性上下文进行建模。在训练过程中,人工神经网络的不同层越来越多地使用每个连续层来构建输入数据,从而对信息产生更抽象的“理解”。当然,只有通过先进的数学方法以及更高的计算能力和更快的图形处理器(GPU)来计算学习过程中的无数步骤,才能使这种深度人工神经网络成为可能。 2013 年,《麻省理工科技评论》将深度学习确定为年度十大突破性技术之一(Hof 2013)。
对于图像识别,“深度卷积神经网络”(一种特定类型的 ANN)已被证明特别有效。与大脑中的视觉皮层类似,这些网络首先从输入数据中提取基本图像特征,如角落、边缘和阴影。然后在多个抽象步骤中,他们独立解决更复杂的图像模式和对象。当这些网络中最好的网络在非医学图像数据库上进行测试时,它们的错误率现在下降到只有百分之几(He et al. 2015)。此外,可以结合不同的网络架构和方法(例如,深度学习与“强化学习”)以根据所提出的问题获得最佳结果。
鉴于这一发展,专家预计医学成像将发生重大变化(Lee 等人,2017 年)。与 1990 年代后期开始在美国引入的以前的 AI 方法不同,特别是用于乳房 X 线照相筛查,但存在很多缺点(Morton 等人 2006;Fenton 等人 2007;Lehman 等人 2015),今天的算法可能会被证明是临床诊断的变革性技术。
Case Study missing?
Start adding your own!
Register with your work email and create a new case study profile for your business.
相关案例.
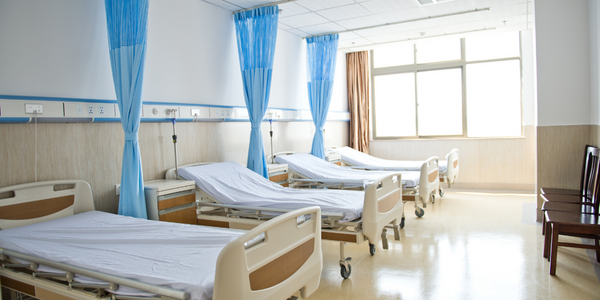
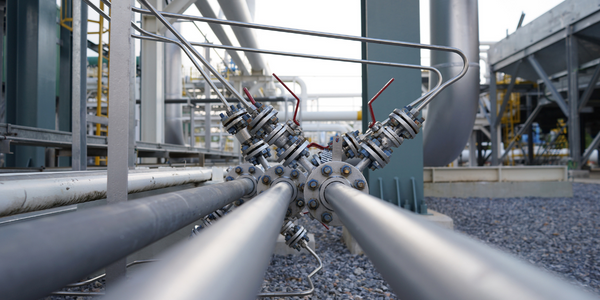
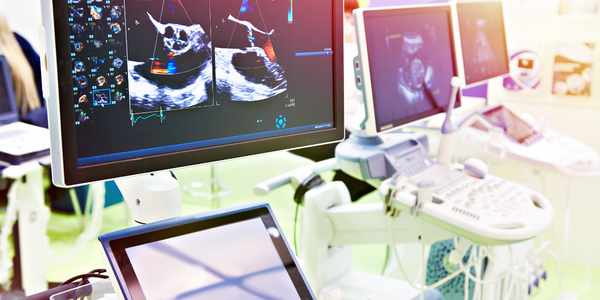
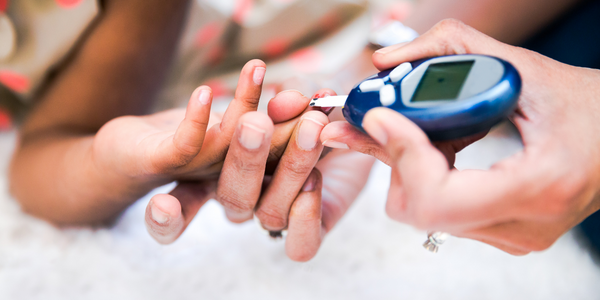
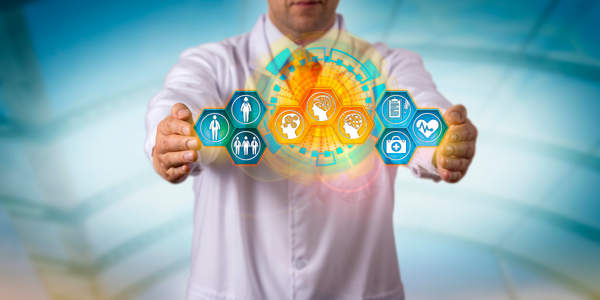