- Analytics & Modeling - Machine Learning
- Analytics & Modeling - Predictive Analytics
- Oil & Gas
- Retail
- Sales & Marketing
- Movement Prediction
- Predictive Maintenance
- Data Science Services
- Training
Audiobooks.com is a flagship audio brand of RBmedia and the world’s largest audiobook publishing company. The company boasts a catalog of more than 250,000 exclusive titles narrated by award-winning actors. Audiobooks.com provides digital content to libraries, schools, and retail markets. As a subscription-based business, the company's primary growth driver is customer retention. Audiobooks.com operates in a competitive market where customers have plenty of alternative providers to choose from, making customer retention a critical aspect of their business strategy.
Audiobooks.com, a leading audiobook publishing company, faced a significant challenge in improving the Cost-per-Circulation (CPC) for digital content and audiobook titles on their platform. The company aimed to predict customer churn using AI to drive more value for their clients and solidify their bottom line. As a subscription-based business, customer retention was crucial for Audiobooks.com. The company operates in a competitive market where customers can easily switch to alternative providers. Any negative experience could prompt a customer to abandon the brand, resulting in a loss of revenue, affecting customer lifetime value (LTV), and increasing the cost of acquiring new customers. The company's goal was to minimize the churn rate to lower CPC, which is calculated as the total cost of their licensing fees vs. the total number of book checkouts. High customer churn negatively impacts book checkouts and makes it difficult for distributors to get government funding. The executive team set a bold goal to track the habits of problem users and proactively make personalized offers to minimize attrition by predicting churn while increasing checkouts.
Audiobooks.com partnered with Provectus to build a more accurate and efficient churn prediction model. Provectus reviewed the existing churn prediction initiatives and set up an evaluation framework to assess data, validate and clarify hypotheses, and check churn prediction models in a consistent and reproducible manner. The team built a dataset consisting of 2.5M user profiles and five years of historical data, such as listening activities, clicks, search and recommendation logs, subscription renewals, and churn events. Several pipelines were developed to produce a deployable churn prediction model. These pipelines enabled quick experimentation, where a new feature generator was added at every successive iteration and its impact on model performance was measured. The final model’s precision and recall did not drop below 95% in different testing scenarios, with its Decision Tree, Random Forest, and CatBoost variants showing 98-99% accuracy. The pipeline built by Provectus allowed the team to see which features influenced churn prediction the most, to come up with more effective strategies to prevent attrition.
Case Study missing?
Start adding your own!
Register with your work email and create a new case study profile for your business.
Related Case Studies.
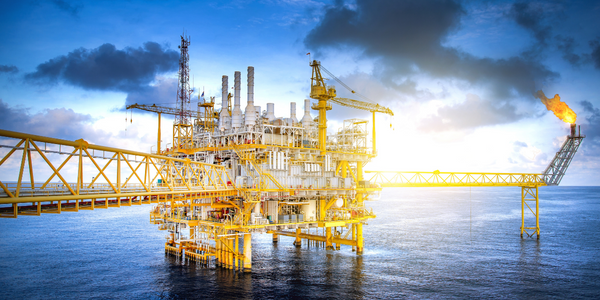
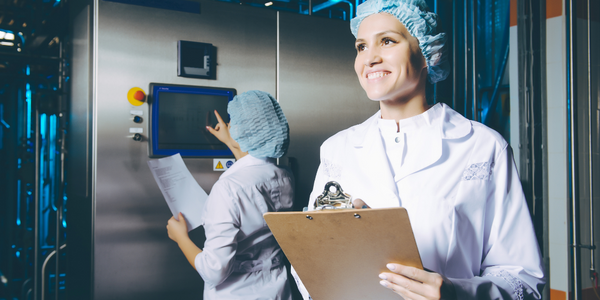
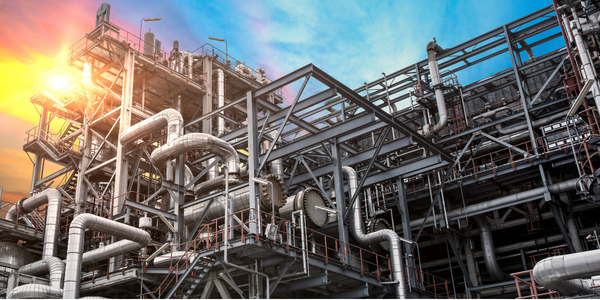