Customer Company Size
Large Corporate
Region
- America
Country
- United States
Product
- H2O Deep Learning
Tech Stack
- Artificial Neural Networks (ANN)
- Deep Neural Networks (DNN)
Implementation Scale
- Enterprise-wide Deployment
Impact Metrics
- Cost Savings
- Digital Expertise
Technology Category
- Analytics & Modeling - Machine Learning
Applicable Industries
- Finance & Insurance
- Telecommunications
Applicable Functions
- Sales & Marketing
- Business Operation
Use Cases
- Fraud Detection
Services
- Data Science Services
About The Customer
The article mentions several companies across industries that rely on H2O for scalable machine learning to detect fraud. These include a U.S.-based payment systems company that handles billions of dollars in payments each month, a global insurance company, a multinational telecommunications provider, and a leading U.S. credit card issuer. These companies turn to H2O because it is highly scalable and delivers superior performance; offers flexible deployment options; works seamlessly in a large scale data sets; and offers a simple interface.
The Challenge
Organizations responsible for fraud prevention are facing a host of challenges at the transaction, account, and network-level to detect fraudulent behavior and suspicious activities. Fraudulent transactions are rare, but costly if they aren’t detected. In the credit card business, for example, third-party fraud accounts for roughly 4 out of every 10,000 transactions. Modeling rare events is difficult, like finding a needle in a haystack. For best results, gather as much data as possible, and use the most advanced techniques available.
The Solution
The solution to the challenge of fraud detection is the use of H2O's Deep Learning technology. This technology uses artificial neural networks (ANN) with multiple hidden layers, also called deep neural networks (DNN). Deep Learning is a rapidly growing discipline that models high-level patterns in data as complex multi-layered networks. Because it is the most general way to model a problem, Deep Learning can solve the most challenging prediction problems. The U.S.-based payment systems company uses H2O Deep Learning for real-time fraud detection. Working with a dataset of 160 million records and 1,500 features, the company’s data scientists use a test-and-learn approach to find the best-performing predictive model.
Operational Impact
Quantitative Benefit
Case Study missing?
Start adding your own!
Register with your work email and create a new case study profile for your business.
Related Case Studies.
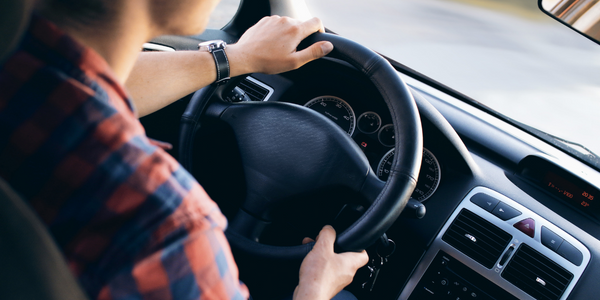
Case Study
Real-time In-vehicle Monitoring
The telematic solution provides this vital premium-adjusting information. The solution also helps detect and deter vehicle or trailer theft – as soon as a theft occurs, monitoring personnel can alert the appropriate authorities, providing an exact location.“With more and more insurance companies and major fleet operators interested in monitoring driver behaviour on the grounds of road safety, efficient logistics and costs, the market for this type of device and associated e-business services is growing rapidly within Italy and the rest of Europe,” says Franco.“The insurance companies are especially interested in the pay-per-use and pay-as-you-drive applications while other organisations employ the technology for road user charging.”“One million vehicles in Italy currently carry such devices and forecasts indicate that the European market will increase tenfold by 2014.However, for our technology to work effectively, we needed a highly reliable wireless data network to carry the information between the vehicles and monitoring stations.”
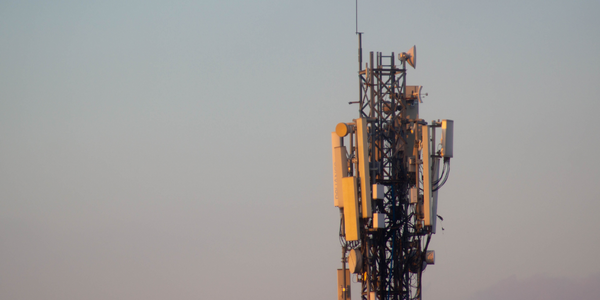
Case Study
Vodafone Hosted On AWS
Vodafone found that traffic for the applications peak during the four-month period when the international cricket season is at its height in Australia. During the 2011/2012 cricket season, 700,000 consumers downloaded the Cricket Live Australia application. Vodafone needed to be able to meet customer demand, but didn’t want to invest in additional resources that would be underutilized during cricket’s off-season.
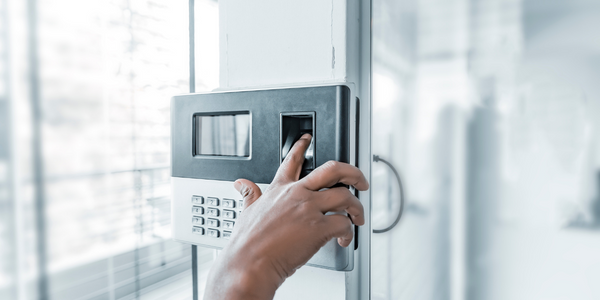
Case Study
SKT, Construction of Smart Office Environment
SK T-Tower is the headquarters of SK Telecom. Inside the building, different types of mobile devices, such as laptops, smartphones and tablets, are in use, and with the increase in WLAN traffic and the use of quality multimedia data, the volume of wireless data sees an explosive growth. Users want limitless Internet access in various places in addition to designated areas.